Generalized Linear Models: With Applications to Linear Models, Logistic and Ordinal Regression, and Survival

Generalized linear models (GLMs) are a powerful class of statistical models that extend the ordinary linear model to accommodate a wider range of response variables. They are used in a variety of applications, including linear models, logistic and ordinal regression, and survival analysis.
GLMs are based on the idea that the response variable follows a distribution from the exponential family. The exponential family is a family of probability distributions that includes the normal, binomial, Poisson, and gamma distributions, among others. The exponential family is characterized by its probability density function, which takes the form:
f(y; θ, φ) = exp[(yθ - b(θ)) / φ + c(y, φ)]
4.6 out of 5
Language | : | English |
File size | : | 13893 KB |
Screen Reader | : | Supported |
Print length | : | 607 pages |
where y is the response variable, θ is the natural parameter, φ is the dispersion parameter, and b(θ) and c(y, φ) are known functions.
The natural parameter θ is related to the mean of the distribution. The dispersion parameter φ is related to the variance of the distribution.
GLMs are fitted by maximizing the log-likelihood function. The log-likelihood function is the logarithm of the joint probability density function of the observed data. Maximizing the log-likelihood function produces parameter estimates that maximize the likelihood of observing the data.
Once a GLM has been fitted, it can be used to make predictions about the response variable. Predictions are made by using the fitted model to estimate the mean of the response variable for a given set of predictor variables.
GLMs are used in a variety of applications, including:
- Linear models: GLMs can be used to fit linear models, which are models in which the response variable is a continuous variable. Linear models are used in a variety of applications, including regression analysis and analysis of variance.
- Logistic regression: GLMs can be used to fit logistic regression models, which are models in which the response variable is a binary variable. Logistic regression models are used in a variety of applications, including classification and prediction.
- Ordinal regression: GLMs can be used to fit ordinal regression models, which are models in which the response variable is an ordinal variable. Ordinal regression models are used in a variety of applications, including ranking and rating.
- Survival analysis: GLMs can be used to fit survival analysis models, which are models in which the response variable is the time until an event occurs. Survival analysis models are used in a variety of applications, including medical research and public health.
The theory of GLMs is based on the idea that the response variable follows a distribution from the exponential family. The exponential family is a family of probability distributions that includes the normal, binomial, Poisson, and gamma distributions, among others.
The exponential family is characterized by its probability density function, which takes the form:
f(y; θ, φ) = exp[(yθ - b(θ)) / φ + c(y, φ)]
where y is the response variable, θ is the natural parameter, φ is the dispersion parameter, and b(θ) and c(y, φ) are known functions.
The natural parameter θ is related to the mean of the distribution. The dispersion parameter φ is related to the variance of the distribution.
GLMs are fitted by maximizing the log-likelihood function. The log-likelihood function is the logarithm of the joint probability density function of the observed data. Maximizing the log-likelihood function produces parameter estimates that maximize the likelihood of observing the data.
Once a GLM has been fitted, it can be used to make predictions about the response variable. Predictions are made by using the fitted model to estimate the mean of the response variable for a given set of predictor variables.
GLMs are fitted by maximizing the log-likelihood function. The log-likelihood function is the logarithm of the joint probability density function of the observed data. Maximizing the log-likelihood function produces parameter estimates that maximize the likelihood of observing the data.
There are a variety of algorithms that can be used to maximize the log-likelihood function. One common algorithm is the iterative weighted least squares (IWLS) algorithm. The IWLS algorithm iteratively updates the parameter estimates until they converge to a maximum.
Once the parameter estimates have converged, the fitted GLM can be used to make predictions about the response variable. Predictions are made by using the fitted model to estimate the mean of the response variable for a given set of predictor variables.
The interpretation of a GLM depends on the type of response variable. For linear models, the interpretation is straightforward. The slope of the regression line represents the change in the mean of the response variable for a one-unit increase in the predictor variable. The intercept of the regression line represents the mean of the response variable when the predictor variable is zero.
For logistic regression models, the interpretation is a bit more complex. The log-odds of the response variable is a linear function of the predictor variables. The log-odds of the response variable is the logarithm of the odds of the response variable occurring. The odds of the response variable occurring is the ratio of the probability of the response variable occurring to the probability of the response variable not occurring.
For ordinal regression models, the interpretation is even more complex. The cumulative log-odds of the response variable is a linear function of the predictor variables. The cumulative log-odds of the response variable is the logarithm of the odds of the response variable being less than or equal to a given category. The odds of the response variable being less than or equal to a given category is the ratio of the probability of the response variable being less than or equal to that category to the probability of the response variable being greater than that category.
For survival analysis models, the interpretation is again complex. The hazard function is a linear function of the predictor variables. The hazard function is the instantaneous rate of failure. The instantaneous rate of failure is the probability of failure at a given time, given that the subject has survived up to that time.
GLMs are a powerful class of statistical models that can be used to model a wide variety of response variables. They are used in a variety of applications, including linear models, logistic and ordinal regression, and survival analysis. GLMs are relatively easy to fit and interpret, making them a valuable tool for data analysis.
4.6 out of 5
Language | : | English |
File size | : | 13893 KB |
Screen Reader | : | Supported |
Print length | : | 607 pages |
Do you want to contribute by writing guest posts on this blog?
Please contact us and send us a resume of previous articles that you have written.
Fiction
Non Fiction
Romance
Mystery
Thriller
SciFi
Fantasy
Horror
Biography
Selfhelp
Business
History
Classics
Poetry
Childrens
Young Adult
Educational
Cooking
Travel
Lifestyle
Spirituality
Health
Fitness
Technology
Science
Arts
Crafts
DIY
Gardening
Petcare
Jon Tyson
Jessica Cornwell
Dr Guy Leschziner
Jacqueline Church Simonds
Kiku Hughes
Kevin Hunter
Trevor Lund
W E Fairbairn
Alan S Gurman
Catherine Belknap
John Irving
Erin Ekins
George E Andrews
Michael Casey
Noha Mellor
John Leo
Samuel N Rosenberg
Akil Palanisamy
Rose Lynn Fisher
Vera Nazarian
Sheila Barton
Alana Chernila
Chris Vines
Nicholas Romanov
Gillian Gill
Tod E Bolsinger
Christopher Gavigan
Alice Oseman
Robert A Sadowski
Nina Lacour
Scott Surovich
Skeleton Steve
Edupan7 Publishing
Alan Murchison
Alice Scordato
Michael Sidgwick
Charles B White
Edward A Tiryakian
Meredith Marsh
Jay Annelli
Alan Porter
Bobby Flay
Dave Heller
J Zoltan
John Riley
John Feinstein
Esther Parry
Mike Martin
Louis Schubert
Robert Heidler
Spencer Wells
Bruce Lee
S A Klopfenstein
Sarah Hendrickx
Alan E Kazdin
Michael Morpurgo
Bradley Efron
Jacqueline Tourville
Marcus Chown
Rand Cardwell
Tim Gallagher
Arthur Fleischmann
Megan Gray Md
John Muir
Bruce J Macfadden
Tina Dreffin
Bill Walker
Olivia Gentile
Joyce Burkhalter Flueckiger
Suze Guillaume
Kiersten White
Caitlin Zaloom
Dan Dipiro
Frances E Jensen
Hervey Garrett Smith
Donald Woods Winnicott
Peg Tyre
Patrick O Sullivan
Jay Revell
Cynthia Clumeck Muchnick
E A Koetting
Jordon Greene
Rehana Jawadwala
Amanda Ford
Aja Raden
David King
Carl Gustav Jung
Magnus Thollander
Morgan Lyle
Alan Shipnuck
Dr Joseph Parent
Stephen Wolfram
Arnold Yates
Florence Williams
David Vine
Will Storr
Christopher Scordo
Massad Ayoob
Dmitry Zinoviev
Larry Wasserman
Doug Gelbert
Contractor Education Inc
Ainsley Arment
Harriet Brown
Jack Nicklaus
Al Yellon
Marc M Triola
Gordon R Dickson
Alan Lightman
Jeff Maynard
Erin Lovelace
Gail Tully
Francesca Lia Block
Kai Nacke
Albert Einstein
Lecile Harris
Howard Greene
Brandy Roon
Jon Emmett
Sir Edmund Hillary
Charlotte Markey
Angelina Wilson
Marsha Wenig
Nick Hughes
Anthonissa Moger
Alan Lawrence Sitomer
Lauren Blackwood
Barbara Oehlberg
George Buehler
Pallas Snider
Po Bronson
Al Barkow
Franz Metcalf
Bryon Powell
Al Marlowe
Rebekah Sack
Michael Domican
Jane Bottomley
George Knudson
Al Ristori
Kardas Publishing
Michael Freedland
Gary R Varner
Amelia Mayer
Dimitri Loose
Grace Lebow
Theodore P Jorgensen
Gregory J Davenport
Joe Bertagna
Kelly Jensen
Margit Ystanes
Anders Halverson
Robin Mckinley
Michael Miller
Matthew Sturm
Bill Friedrich
We Fairbairn
Sandy Lindsey
Jerry Robeson
Noel St Clair
Teresa Mccallum
Janet Houser
Aileen Evans
Gary Smith
Bryan Mann
Timm Woods
Karen Levin Coburn
Mykel Hawke
Ged Jenkins Omar
Chris Klug
George Plimpton
Jonathan Gottschall
Douglas G Altman
Jonathan White
James A Michener
Don Casey
Denise Alvarado
Truman Everts
G E R Lloyd
Lj Andrews
Barbara Scott
Miles Mckenna
John Bird
Dave Whitson
Collins Easy Learning
David Crabtree
Kareem Abdul Jabbar
Aiva Books
Andrew Rowe
Layla Moon
Alan E Sparks
Daniel C Okpara
Alan Burdick
Dawn Sakamoto Paiva
Alan Garner
Josh Kilmer Purcell
Mackenzi Lee
Jeremy Miles
Jessica Battilana
Michael Gray
Al Strachan
Joe Clement
Jay H Lefkowitch
Mendocino Press
Jane Poynter
Matt Mosher
Elizabeth Thompson
Al Macy
Penney Peirce
Christos A Djonis
Mark Occhilupo
Shoshana Levin Fox
Snap Summaries
Shaun Tomson
Nilakantasrinivasan J Neil
Paramahansa Yogananda
R A Salvatore
Gina Guddat
Hiro Ainana
Alan Gelb
Lee Carroll
Gill Rapley
Tom Shachtman
Takuan Soho
Lex Croucher
Helen Hollick
Jessica Cunsolo
Joe Berardi
Tonya Johnston
Deborah Harkness
Aidan Comerford
Hammad Fozi
Gavin Francis
Courtney Macavinta
Juno Roche
Zita West
Tenth Edition Revised Kindle Edition
John T Hansen
Kevin B Shearer
Habeeb Quadri
Taquetta Baker
Andrew Blum
Jill Homer
Andre Agassi
Andrew Jamieson
Robert E Stake
Michael Shermer
Eden O Neill
Sam J Fires
Steve Michalik
Farlex International
Ai Publishing
Hope Smith
Brian Mills
Steven Horak
Amye Archer
Satya Sundar Sethy
Harold Klemp
Shing Tung Yau
Anne Enright
Roy Dittmann
David D Burns
Dr David Geier
Melissa Bashardoust
Gayle Peterson
Howard Hill
Jackson Katz
Sara Hanover
Leif K Karlsen
Alan Vermilye
Betsy Tobin
Sonia Nazario
Kim Stanley Robinson
Martin Erwig
Kerry Diamond
Ann Hagedorn
Terry Breverton
Kevin Williams
Dennis Jarecke
Celeste Jones
I Johnson
Judith Garrard
George M Fredrickson
Pavel Tsatsouline
Cat Warren
Alan R Hall
Bill Parisi
Martin J Pring
Charles J Thayer
G H Hardy
Alana Mclaren
Aidan Dodson
Nassim Nicholas Taleb
Graham Bower
Craig Childs
Rosemarie Anderson
Jan Smith
Beata Bliss Lewis Md
Saul Rip
Judy Jewell
Anna Hickey Moody
Alan C Acock
Jakob Tanner
James Willard Schultz
Alastair Humphreys
Aphra Behn
Alan S Kaufman
Robert L Kelly
Elaine Howard Ecklund
Yang Jwing Ming
Micky Ward
Hannah Ewens
Melinda Rushe
Lexie Scott
Alex Light
Benjamin Johnston
Al Roker
Aidan Chambers
Juliet Marillier
Chris Bowers
12th Edition Kindle Edition
Bianca Smith
Lo Bosworth
James Witts
Aileen Nielsen
Jim Steenburgh
Al Desetta M A
The Rogue Hypnotist
Mark Drolsbaugh
M K Hume
Robert M Emerson
Aimee Aristotelous
Arnold Haultain
Alan Graham
E Cheng
Rick Bass
Conor Daly
Madeline Milne
Martin Rooney
Linda G Morris
Vernon Coleman
John J Miller
United States Government Us Marine Corps
Mark Goulston
Donna Morang
Ilan Dvir
Jacqueline Fitzpatrick
Mark Booth
Terry Funk
Molly Absolon
Kate Brian
Antipodean Writer
Carol Tuttle
David A Sousa
Mariam N Ottimofiore
Alan Margot
Vasilis Konstantinos Giontzis
Orson Scott Card
Jill Stamm
Susan B Lovejoy
Nancy Hughes
Christopher Willard
Stephen M Camarata
Vanessa Ogle
Bob Plott
William A Kaplin
Joanne Webb
Rene D Zweig
Jenni Hicks
Percy Boomer
Terry L Duran
Margaret J Wheatley
Peter Irvine
Robert Macfarlane
Princess Her
Mike Warren
Akwaeke Emezi
Douglas T Hall
George Monbiot
Belinda Luscombe
Thomas Metzinger
Lisa Marasco
Dorothy H Crawford
Stephanie Izard
Matthew Mcbee
Anahad O Connor
Alan Booth
Frank E Harrell Jr
Peter Hathaway Capstick
Kate Bettison
Alan Rouse
Letisha Galloway
John H Moore
Paul G Schempp
Laurie Pickard
Jerry Robertson
Jacob K Olupona
Helena Clare
Bob Mayer
Kerstin Gier
Steven H Strogatz
Carla Schroder
Bettina Gordon Wayne
Emily Harris Adams
S Connolly
David F Kelly
Roxy And Ben Dawson
Ken Denmead
Clyde Fitch
Billy Walsh
Gary B Ferngren
Andy Zipser
Jamie Baulch
William Foote Whyte
Daphne Rose Kingma
Ahmed Osman
Ndeye Labadens
Samuel Carbaugh
Ron L Deal
Vishwesh Bhatt
Constance Classen
Publishdrive Edition Kindle Edition
Amy Edelstein
Leigh Sales
Linda Welters
Erica Westly
Wayne Stewart
Isabella Morris
Jane P Gardner
Michal Stawicki
Alan Watts
William Harding
Markus Rex
Mark Harris
Jeanne Faulkner
Dylan Alcott
Steven Trimble
Anne Dachel
Alan Hearnshaw
Celeste Headlee
Michael D Rich
Len Sperry
Mike Rothschild
Andy Adams
Martin Luther King Jr
Kristine Barnett
Nick Lyons
Emeran Mayer
Aileen Erin
Gordon H Orians
Peter Ballingall
Toby Wilkinson
Lisa Newton
David Jason
Rob Casey
Cindy Hudson
Rebecca Jones
Mary Basick
Alfred S Posamentier
Al Pasha
Lisa Kenney
Al Owens
Thomas Kurz
Howard Maxwell
Amelia Simmons
Mel Thompson
Damon Lee
Julie Bradley
Natazha Raine O Connor
James Gleick
Sara Wickham
Alaina Talboy
Janice Selekman
Clint Emerson
Helen Palmer
Original Edition Kindle Edition
David Roberts
James F Boswell
Ken Schultz
Thubten Chodron
Mista Nove
Lingo Mastery
Douglas Noll
Andrea Travillian
Deitra Leonard Lowdermilk
Dusty Rhodes
Timothy C Smith
Eddy Starr Ancinas
J R Haseloff
Jay Speight
Richard Pears
Aitzaz Imtiaz
Michael W King
Pantea Kalhor
Al Sweigart
Katharine Graves
Gary Player
Christopher Hellman
Kidada Jones
Zondervan
Brenda Nathan
Steve Moore
Sophia Joy
Dean Burnett
Light bulbAdvertise smarter! Our strategic ad space ensures maximum exposure. Reserve your spot today!
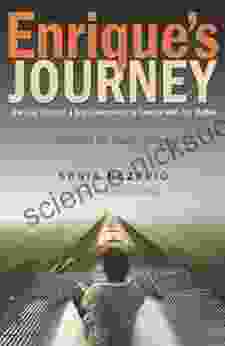

- John ParkerFollow ·11.1k
- Samuel WardFollow ·14.8k
- Orson Scott CardFollow ·18.6k
- Junot DíazFollow ·15k
- José SaramagoFollow ·13.2k
- Garrett BellFollow ·4.3k
- Dave SimmonsFollow ·4.4k
- Dwayne MitchellFollow ·16k
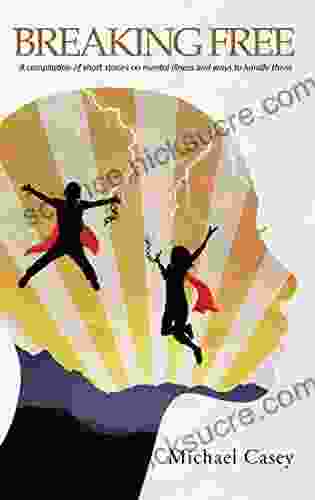

Compilation of Short Stories on Mental Illness and Ways...
Mental illness is a serious issue that affects...
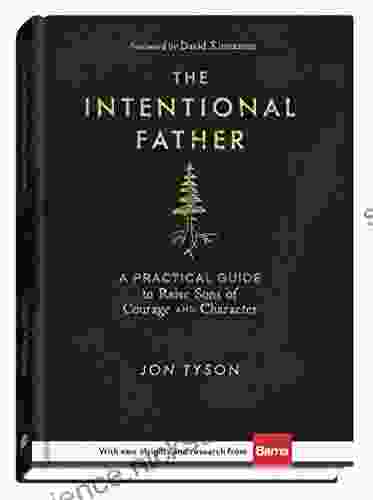

The Practical Guide to Raising Courageous and...
As parents, we all want...
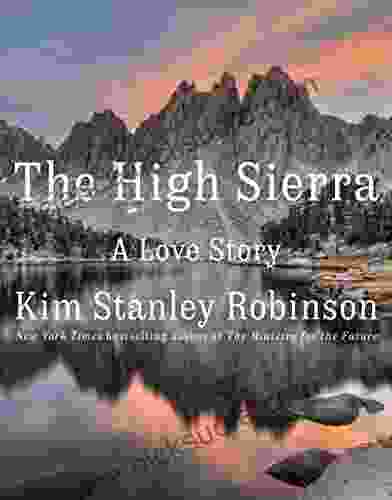

A Journey to Remember: The High Sierra Love Story of...
Prologue: A Wilderness Encounter Beneath...
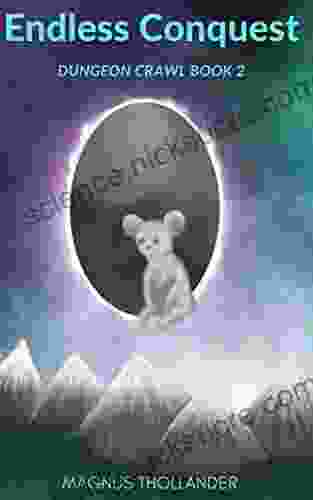

Endless Conquest: Embark on an Immersive Dungeon Crawl in...
Endless Conquest is a captivating LitRPG...
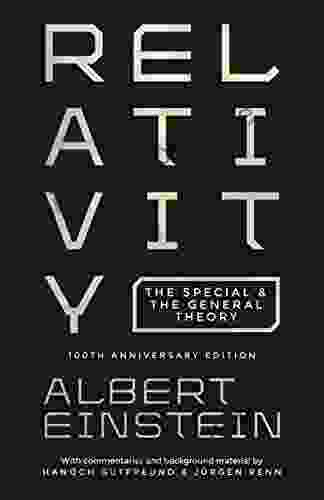

The Special and the General Theory 100th Anniversary...
The year 2015 marks a...
4.6 out of 5
Language | : | English |
File size | : | 13893 KB |
Screen Reader | : | Supported |
Print length | : | 607 pages |